On indirect AI bias, ethics, and asking the wrong question
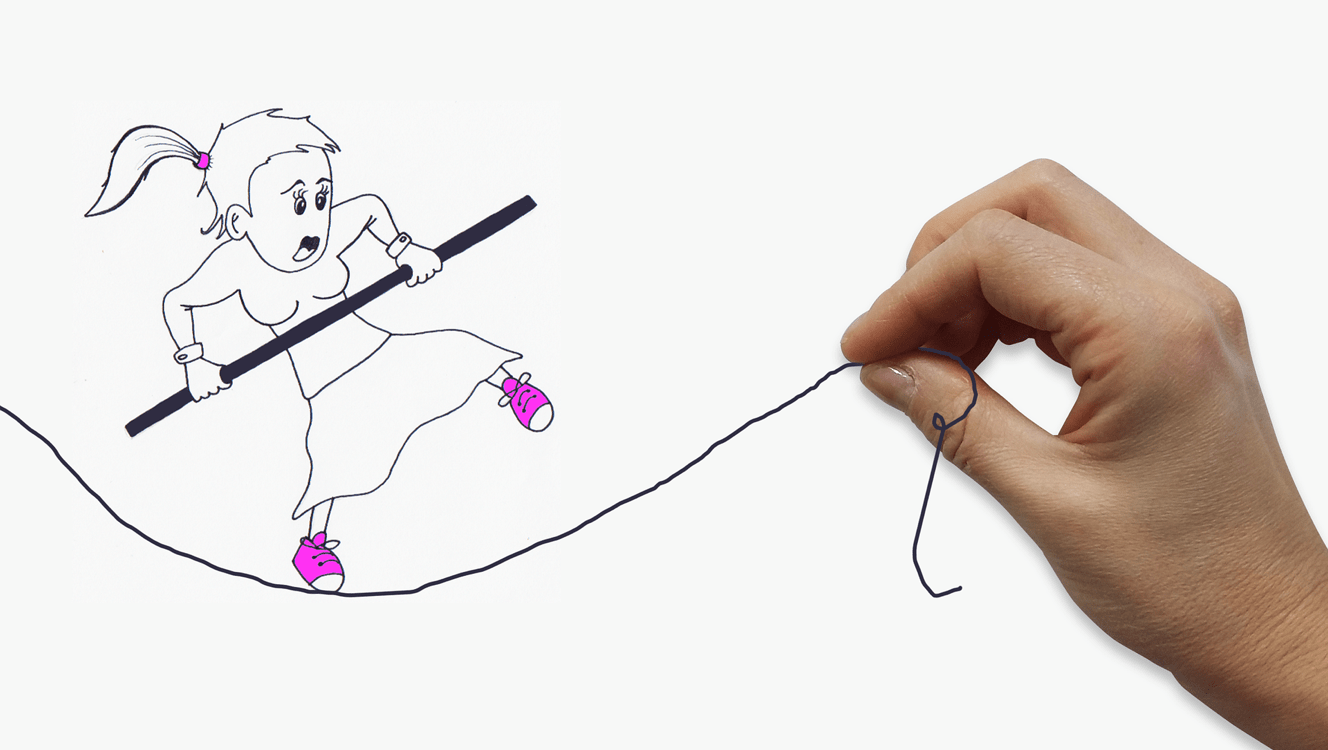
Since the use of artificial intelligence (AI) has become ubiquitous today, there are proportionally more opportunities for this amazing piece of tech to fail. And one area where AI still fails is delivering the same level of service to all, regardless of their race, gender, and other characteristics.
Face recognition apps frequently fail to correctly interpret certain types of faces. On one occasion, New Zealand’s passport robot prompted a man of Asian descent to open his eyes. On another occasion, Google’s face app mistakenly labeled a photo of black people as “gorillas.” Black folks fear that self-driving cars may have trouble identifying darker-skinned pedestrians. So AI bias could put lives at risk.
Data scientists say that’s because some algorithms are trained on unrepresentative or otherwise problematic datasets. This could lead us to think that, if we use the right data, we’ll get a bias-free outcome. But the problem is not limited to rogue datasets. Rather, it sometimes lies with the question we want that data to answer. And this problem is much harder to identify and fix.
Asking the wrong question
Do you remember the conversation between the pan-dimensional beings and the supercomputer from The Hitchhiker’s Guide to the Galaxy?
– Deep Thought, do you have…
– An answer for you? Yes, but you’re not gonna like it.
– It doesn’t matter, we MUST know it.
– All right! The answer to the ultimate question of life, the Universe, and everything is… forty-two! [Everyone gasps.]It would have been simpler, of course, to have known what the actual question was.
– But it was THE question! The ultimate question for everything!
– That’s not a question. Only when you know the question, will you know what the answer means.
– Give us the ultimate question then!
– I can’t…
Just like Deep Thought in Douglas Adams’s sci-fi story, the computer in real life can’t help you frame the question. At the same time, framing the question in an unbiased and ethical way is crucial if you don’t want to end up with a racist or sexist system.
Indirect AI bias
Let’s say you set out to build an AI application and take care not to incorporate signals like race or gender, because they may skew your results. Yet, you use signals that tend to correlate strongly with race and gender, shooting yourself in the foot and introducing bias anyway.
Take criminal justice, for instance. Many courts across the United States use risk of recidivism assessment tools to help decide on the size of bail or how long one gets to spend in jail. Yet, the resulting risk scores are often skewed to the detriment of black defendants, researchers say. ProPublica analysis shows that the system mistakenly labels black defendants high-risk just as often as it mistakenly labels white defendants low-risk.
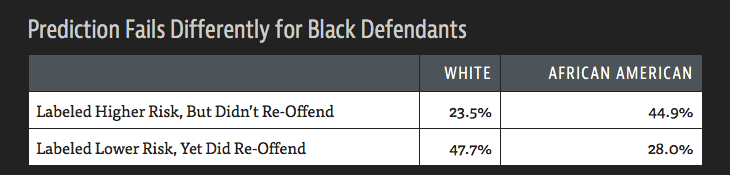
The answer, perhaps, lies in the 137 questions included into one of the most popular risk assessment tools used in the US. Race is not one of them, but questions like, “How often do you have barely enough money to get by?” and, “Is it easy to get drugs in your neighborhood?” could be proxy to asking about one’s race.
Luckily, judges say they don’t blindly trust software-produced risk scores. Yet ProPublica reports situations when black defendants got rather harsh sentences for petty crimes they committed. Could their high risk scores be to blame?
But let’s consider another domain, which is hiring. A scandal broke out about a year ago when it was found out that Amazon’s internal candidate screening system was favoring men over women. Since the gender attribute was not incorporated into the system, it took Amazon quite a lot of brain-wracking to get to the bottom of this. Turns out, the algorithm had been learning off of 10 years of historical data and picked up on a pattern where the word “women’s” (as in “women’s college” or “women’s league”) on a resume did not correlate with a successful hire.
Eventually, Amazon said their recruiters hardly used the scores, so the company ended up retiring the app. But what Amazon’s example illustrates is that there could be specific language somewhere in those oodles of data that correlates with masculine or feminine identity. And it may be very hard to catch.
AI ethics
Even before creating machine learning-powered software, engineers are often faced with a dilemma: if they include certain signals that correlate strongly with, say, race or gender, that will make their analysis more reliable, but could also make their system biased.
So, whether you’d be willing to do that or not is a question of ethics, really. Many in the data science community have been talking about the need for ethical guidelines in the development and the application of AI.
Many say that understanding your responsibility as a data scientist should start in the classroom. The National Academies of Sciences, Engineering, and Medicine in the US has proposed the Data Science Oath for data scientists to take. It is very close to the Hippocratic Oath and has a clause on being aware of AI bias, among other things:
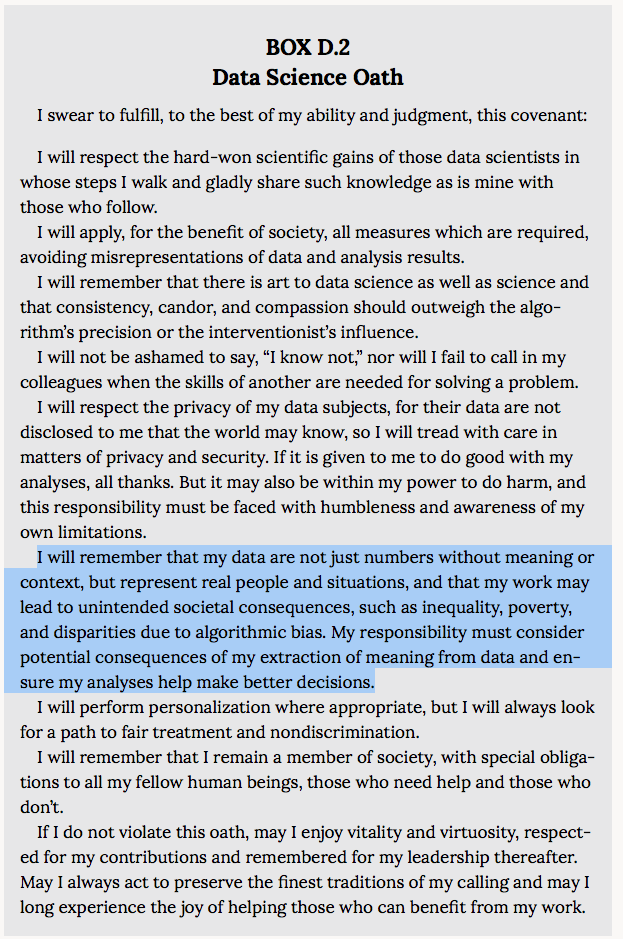
At the same time, AI ethics isn’t about AI bias only. It’s a broader collection of topics ranging from the use of AI for military and surveillance to discussions on AI rights in a church-like context.
Transcending the blind spots
On the upside, there are positive developments. As the global society moves towards greater inclusion, software developers begin to take note of the individuals that were previously invisible to them.
For instance, in the past, women were more at risk of getting sick at work or dying in a car crash because neither crash tests, nor healthy office temperature measurements included data on women.
Today, this has changed. And the changes are fueled, in part, by a shift in the perception of “the average human” in the society. Just recently, German psychologists compared the drawings of kids who were asked to “draw a person” in 1977 and 2015. Back in 1977, 70% of drawings were of men and only 18% were of women. In 2015, the proportion shifted to 40% and 47% respectively, the remaining drawings being impossible to place. Besides, depictions of female humans have become more unapologetically feminine over time, researchers say.
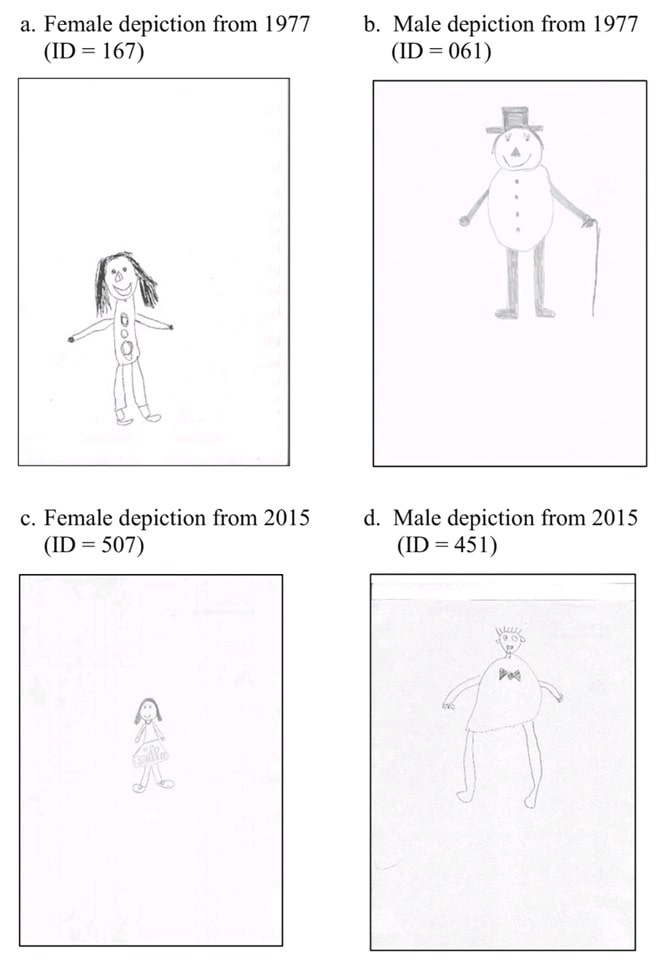
In addition to the shifting stereotypes, there is also more awareness of AI bias as a problem now. Today we have initiatives like Joy Buolamwini’s Algorithmic Justice League that help companies test for AI bias and run exposés of malpractices by corporate entities.
In conclusion
AI bias is a potential for an algorithm to produce incorrect outcomes based on the flawed data in its system. While it may be relatively easy to point a finger at a clearly unrepresentative dataset, it’s harder to work out the ethical question of whether certain systems should be built in the first place. Some systems based on historical data are doomed to amplify people’s biased attitudes, so we should be careful about which questions we want AI to answer – not everyone may like what they get.
Related Blogs
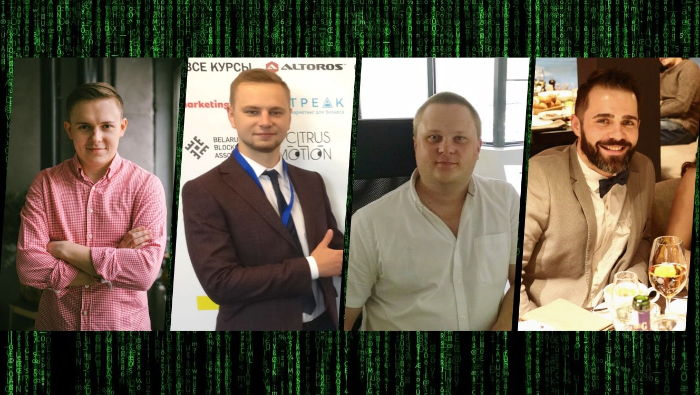
Discussion: Do Humans Expect Too Much of AI?
Read Article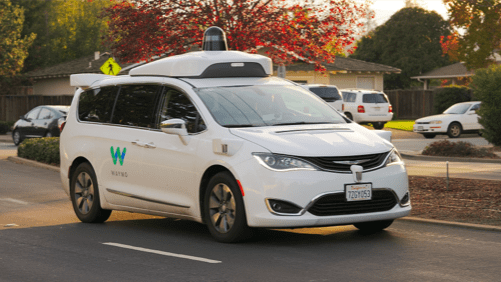